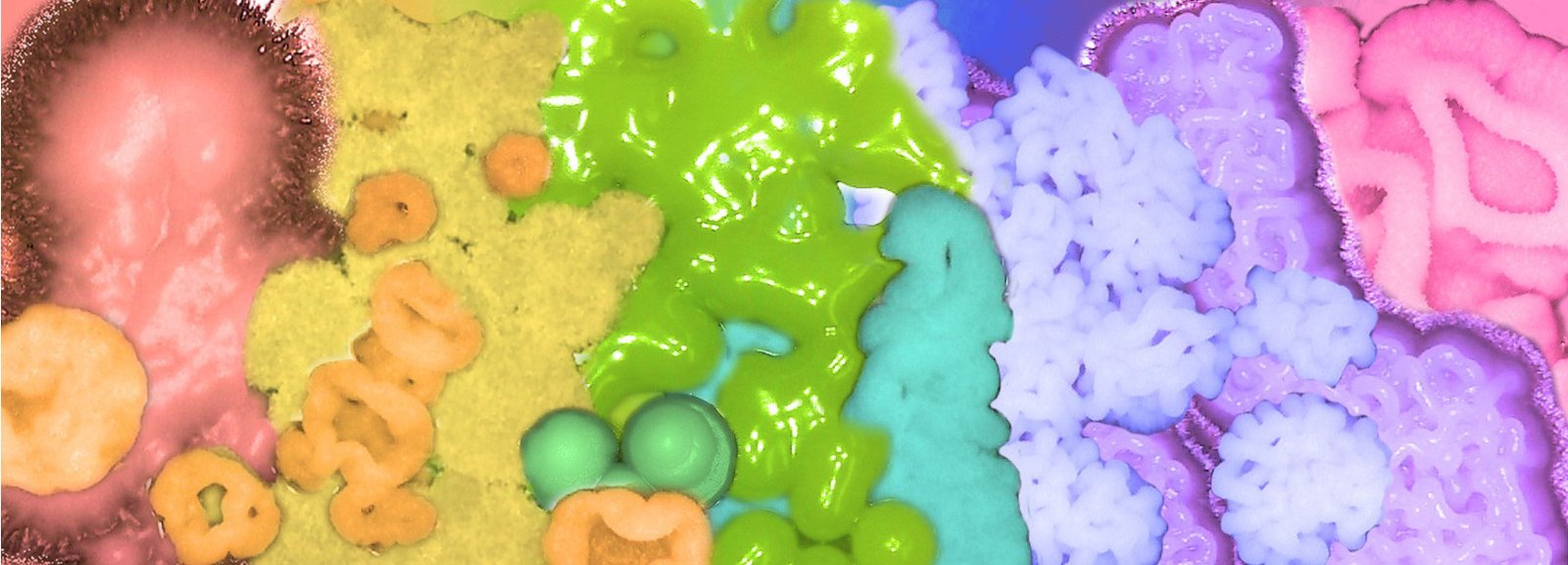
Surprising Patterns Unearthed – Study Debunks Traditional Views on Yeast Evolution
-
by Anoop Singh
- 22
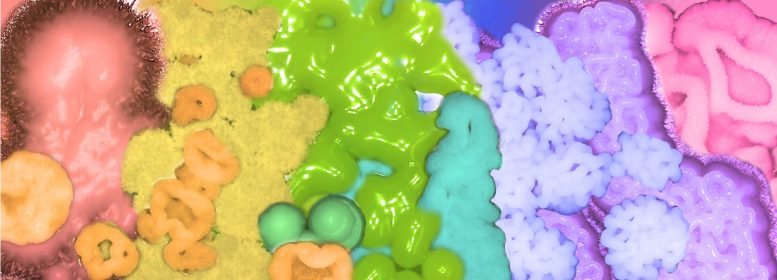
A study reveals new insights into yeast evolution through AI analysis of over 1,000 strains, challenging old paradigms and providing a comprehensive dataset for advancing research in several scientific domains. Yeast colonies (artificially colored). Credit: UNC Charlotte
University of North Carolina at Charlotte Assistant Professor of Bioinformatics Abigail Leavitt LaBella has co-led an ambitious research study — published in the prestigious journal Science — that reports intriguing findings made through innovative artificial intelligence analysis about yeasts, the small fungi that are key contributors to biotechnology, food production, and human health.
The findings challenge accepted frameworks within which yeast evolution is studied and provide access to an incredibly rich yeast analysis dataset that could have major implications for future evolutionary biology and bioinformatics research.
LaBella, who joined UNC Charlotte’s Department of Bioinformatics in the College of Computing and Informatics as an assistant professor and researcher at the North Carolina Research Campus in 2022, conducted the study with co-lead author Dana A. Opulente of Villanova University. They collaborated with fellow researchers from Vanderbilt University and the University of Wisconsin at Madison, along with colleagues from research institutions across the globe.
This is the flagship study of the Y1000+ Project, a massive inter-institutional yeast genome sequencing and phenotyping endeavor that LaBella joined as a postdoctoral researcher at Vanderbilt University.
“Yeasts are single-celled fungi that play critical roles in our everyday lives. They make bread and beer, are used in the production of medicine, can cause infection, and as close relatives to animals have helped us learn about how cancer works,” said LaBella. “We wanted to know how these small fungi have evolved to have such an incredible range of functions and features. With the characterization of over one thousand yeasts, we found that yeasts do not fit the adage ‘jack of all trades, master of none.’”
Study Findings and Implications
This study contributes to basic understanding of how the microbes change over time while generating more than 900 new genome sequences for yeasts — many of which could be leveraged in biofungal fields such as agricultural pest control, drug development, and biofuels production.
LaBella and her co-authors — through an artificial intelligence-assisted, machine-learning analysis of the Y1000+ Project’s dataset comprising 1,154 strains of the ancient, single-cell yeast Saccaromycotina — attempted to answer an important question. That is: Why do some yeasts eat (or metabolize) only a few types of carbon for energy while others can eat more than a dozen?
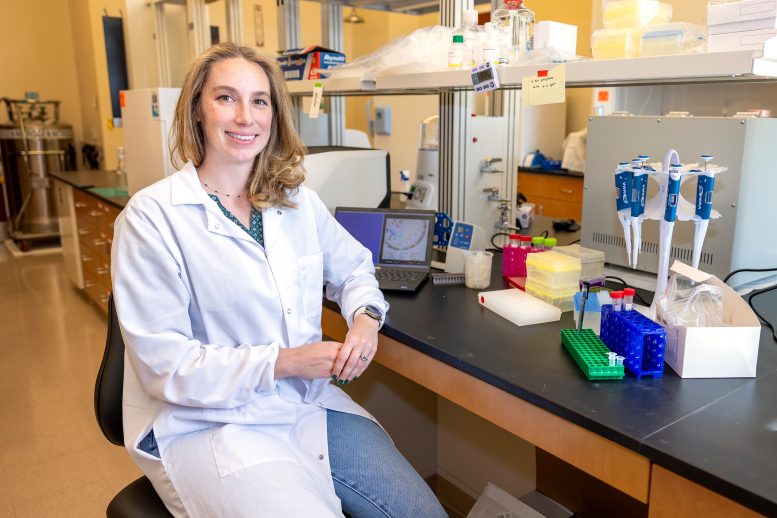
Abigail Leavitt LaBella. Credit: UNC Charlotte
The total number of carbon sources used by a yeast for energy is known in ecological terms as its carbon niche-breadth. Humans also vary in their carbon niche breadth — for example, some people can metabolize lactose while others cannot.
Evolutionary biology research has supported two key overarching paradigms about niche breadth, the phenomenon explaining why some yeast organisms (“specialists”) evolve to be able to metabolize only a small number of carbon forms as fuel while others (“generalists”) evolve to be able to consume and grow on a broad variety of carbon forms. One of these paradigms illustrates that being a generalist comes with certain trade-offs compared to being a specialist. Notably, in the latter case, the ability to process a wide range of carbon forms comes at the expense of the yeast’s capacity to process and grow on each carbon form efficiently. The second is that these yeast specialists and generalists evolve to fit either profile due to the combined effects of different intrinsic traits of their respective genomes and different extrinsic influences based on the varying environments in which yeast organisms exist.
Challenging Existing Paradigms
LaBella and her colleagues found ample evidence supporting the idea that there are identifiable, intrinsic genetic differences in yeast specialists versus generalists, specifically that generalists tend to have a larger total number of genes than specialists. For example, they found that generalists are more likely to be able to synthesize carnitine, a molecule that is involved in energy production and often sold as an exercise supplement.
But unexpectedly, their research found very limited evidence for the anticipated evolutionary trade-off of a yeast’s ability to process many forms of carbon coming at the expense of its ability to do so efficiently and grow accordingly, and vice versa.
“We saw that the yeasts that could grow on lots of carbon substrates are actually very good growers,” said LaBella. “That was a very surprising finding to us.”
While the findings of this specific experiment and the innovative machine-learning mechanisms used in its analysis could have major implications for bioinformatics, ecology, metabolics, and evolutionary biology, the publishing of this study means that the Y1000+ Project’s massive compendium of yeast data is now available for scholars worldwide to use as a starting point to amplify their own yeast research.
“This dataset will be a huge resource going forward,” said LaBella.
Reference: “Genomic factors shape carbon and nitrogen metabolic niche breadth across Saccharomycotina yeasts” by Dana A. Opulente, Abigail Leavitt LaBella, Marie-Claire Harrison, John F. Wolters, Chao Liu, Yonglin Li, Jacek Kominek, Jacob L. Steenwyk, Hayley R. Stoneman, Jenna VanDenAvond, Caroline R. Miller, Quinn K. Langdon, Margarida Silva, Carla Gonçalves, Emily J. Ubbelohde, Yuanning Li, Kelly V. Buh, Martin Jarzyna, Max A. B. Haase, Carlos A. Rosa, Neža ČCadež, Diego Libkind, Jeremy H. DeVirgilio, Amanda Beth Hulfachor, Cletus P. Kurtzman, José Paulo Sampaio, Paula Gonçalves, Xiaofan Zhou, Xing-Xing Shen, Marizeth Groenewald, Antonis Rokas and Chris Todd Hittinger, 26 April 2024, Science.
DOI: 10.1126/science.adj4503
A study reveals new insights into yeast evolution through AI analysis of over 1,000 strains, challenging old paradigms and providing a comprehensive dataset for advancing research in several scientific domains. Yeast colonies (artificially colored). Credit: UNC Charlotte University of North Carolina at Charlotte Assistant Professor of Bioinformatics Abigail Leavitt LaBella has co-led an ambitious research…
A study reveals new insights into yeast evolution through AI analysis of over 1,000 strains, challenging old paradigms and providing a comprehensive dataset for advancing research in several scientific domains. Yeast colonies (artificially colored). Credit: UNC Charlotte University of North Carolina at Charlotte Assistant Professor of Bioinformatics Abigail Leavitt LaBella has co-led an ambitious research…